Predict to prevent
In this project, we investigate specific parameters from big data in ‘Malvern Panalytical’s line of Zetium XRF wavelength dispersive spectrometers’. These perform analyses concerning material consistency and composition and they are key instruments for practically all domains, from food to medicine and construction.
Malvern Panalytical has a very clear purpose in mind: to be able to predict three weeks in advance if a failure occurs in their machines. This should give their service field engineers sufficient time to perform just-in-time maintenance without disrupting active duty of the machine. Key to this purpose are the data which the systems collect while they are operative.
Topics
Predictive maintenance, machine learning, intelligent diagnostics, data management
Program objectives
Adopting the CRISP-DM methodology, we will deliver two end results:
- A selection of key parameters which should be monitored, based on their relevance and importance to predict malfunctions. This includes substantiated thresholds for these parameters.
- A validated methodology and data processing pipeline to generate models with predictive value for the use case, including generated models.
These results will be validated through a software prototype which can process data from machines in situ and generates timely predictions on possible malfunctions.
Partners
Saxion (Ambient Intelligence), Malvern Panalytical
Duration
01-05-2022 – 30-04-2023
More information
Contact Jeroen Linssen:
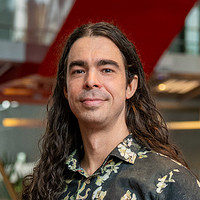
Financing
This project is financed by TechForFuture.