XAIDHA: Explainable AI voor de Digitale Hulpmiddelen-Assistent
Healthcare workers are unaware of the existence of healthcare technology and find it challenging to determine when a client could benefit from specific healthcare technology. The inclusion and exclusion criteria have been shared during a training session or a message on the intranet. However, these are not retained. The current efforts within Nedap Healthcare include a pilot that has been conducted on a limited scale to determine the value of a decision aid for healthcare technology. This has proven successful, and therefore, Nedap wants to develop a generic decision aid solution: one that works for all healthcare organizations and all proven forms of healthcare technology. To develop a solution on this scale, there is a need for knowledge and expertise in explainable AI, for which this collaboration with Saxion has been started. With the outcome of this project, the client population will be analyzed based on the inclusion and exclusion criteria for each form of healthcare technology. As a result, healthcare technology can be deployed effectively in suitable client cases.
Topic
Explainable AI, machine learning, healthcare
Program objectives
The end product of this project will be a software tool capable of providing reliable, personalized suggestions for the deployment of healthcare technology. Ambient Intelligence is collaborating with Nedap Healthcare to facilitate targeted innovations in the field of data analysis using artificial intelligence (AI). Central to this vision is that a substantiated suggestion for a personalized choice for specific healthcare technology can be given based on available data from the Electronic Client Dossier (ECD). This intended solution is encapsulated in the term ‘Digitale Hulpmiddelen-Assistant’ (DHA). This will support the healthcare worker by providing a suggestion for proven healthcare technology when a client meets a certain profile based on the Omaha system for care description. This profile is formed based on indicators (inclusion and exclusion criteria) from the data of the ECD. Based on extensive data analyses using AI techniques such as decision trees, random forests, and possibly more complex algorithms, it will be investigated how these indicators can be traced by a model based on available data. A principle that we adhere to here is that these methods must be transparent – our research for this takes place in the field of so-called explainable AI (XAI). This concept revolves around end users being able to see or follow the reasoning of the generated models, so that the models are understandable and can be deployed responsibly.
Partners
Nedap Healthcare
Duration
01-10-2023 to 30-09-2024
More information
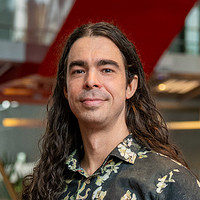
Financiering/Financing
This project is financed by TFF